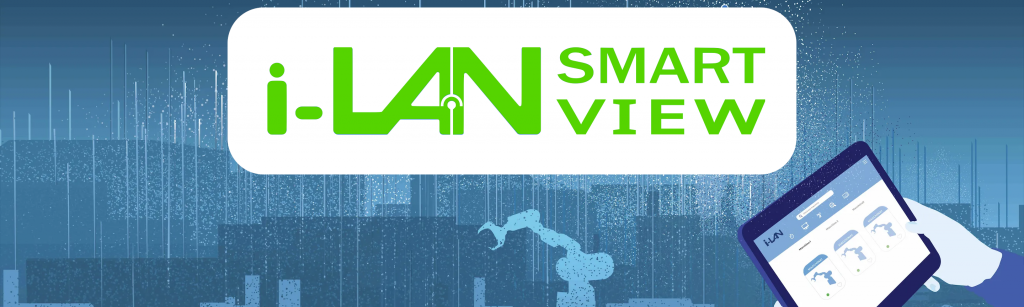
Our Smart View solutions can overcome the limitations of classic vision systems.
They are specific to each customer and application context, minimizing the cost of integration with existing lines and offering broad parameterization flexibility
They adopt advanced Machine Learning techniques, offering resilient performance to environmental changes and the ability to improve over time
They are based on commercial hardware products of recognized reliability, being cost-effective and flexible according to the operating environment
Machine Learning
The system is trained to distinguish between positive and negative situations through a preliminary learning phase, the duration of which depends on the availability of case histories and the complexity of the features to be recognized
Thanks to the learning phase, the system is also able to recognize situations never previously observed, thus adapting to changing operational contexts
The Machine Learning models adopted are directly derived from recognized mathematical models widely adopted by the scientific community
The learning phase can be repeated cyclically to refine system performance or to adapt automatically to changes in the production environment
Multiple recognition models can coexist simultaneously, so the functionality of Smart View stations can be expanded over time
Flexibility and modularity
The control software can be installed on both common Panel PCs and dedicated industrial PCs
The computing resources required are based on the adoption of NVidia Quadro GPU modules or cards.
Ordinary IP cameras with interchangeable optics are sufficient
The system can automatically coordinate complex lighting systems in order to best highlight the features to be recognized
Via industrial communication bus, the SmartView system is configured to interact directly with the line controller to receive trigger events and return inspection outcomes
The User Interface is customized according to the customer’s needs and allows changing working parameters and enabling different features to be recognized.
Conclusions
Introduced solutions based on “machine learning” have been shown to adapt well to a manufacturing environment where materials and defects are characterized by wide variability in shape and position.
The integration of the Smart View system with the existing station did not involve any particular disruption in production, as did the fine-tuning of the system
Recognition performance can be further improved with new training campaigns, without the need for any upgrade of system software or hardware components
The features to be recognized can be expanded with not particularly invasive software upgrades